Fighting Financial Fraud with Splunk
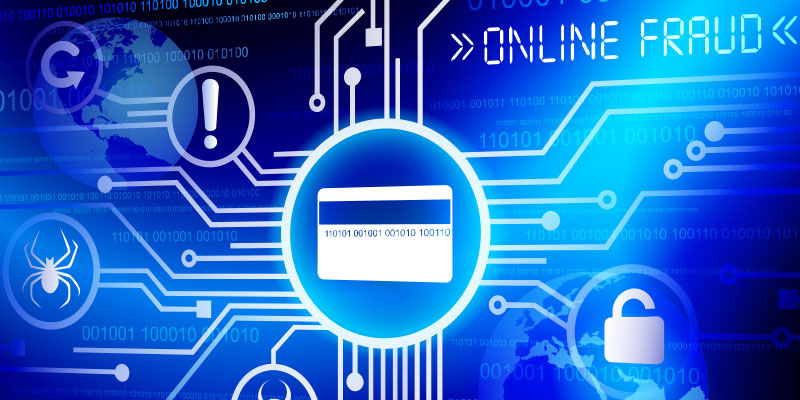
Introduction
It comes as no surprise that as the banking industry is increasing its online presence, financial organizations are making fraud detection and prevention a top priority. Fraud can have a significant impact to organizations both financially and operationally. Of course, in addition to large monetary losses, damage to reputation and customer relations can also occur that further highlight the impact of fraud.
The ability for organizations to perform advanced analytics on their data is necessary to recognize and respond to patterns of fraud. Simply stated, quicker fraud detection is essential to minimizing loss.
Splunk to the Rescue!
As Splunk states, “Fraud, theft, and abuse detection and prevention is a big data challenge, especially as business moves online. Patterns of internal or external fraud often lie in the massive amounts of unstructured machine data and log files generated by your business applications and systems.”
In this blog, I discuss how Function1 has assisted customers with using Splunk for fraud detection and analytics. We will outline some example use cases built in Splunk and highlight other features of Splunk that we have utilized in the fight against financial fraud.
Fraud Detection Use Cases
Much like in cyber security, Splunk can be used for a wide variety of anti-fraud use cases. Although different organizations will have their own unique anti-fraud challenges to address, there are common use cases across the banking and financial industries. Here are some examples of use cases that Function1 has implemented using Splunk for some of our customers.
Fraudulent Membership and Card Applications
- Applications started and completed in a short period of time
- Velocity use cases:
- Many accounts created from one IP/device
- Many accounts sharing the same personal information
- Email addresses with suspicious/invalid email domains
- Geo-location focused use cases
- Integrations with external or third party fraudster databases
Account Takeover
- Unusually high value or number of transfers or withdrawals.
- Velocity use cases:
- Many accounts accessed via one IP/device
- One account accessed via many IPs/devices
- Profile and address changes followed by:
- Transfers or withdrawals
- New Credit/Debit Card requests
- Blacklist/Lookup based use cases:
- Banned device IDs
- Suspicious IP domains/providers
- Brute force use cases – excessive amount of failed/lockout events followed by a successful login
- Geo-location focused use cases
- Integrations with external or third party risk scoring systems
Functionality and Adaptation
Beyond just use case development, Splunk can also be used to provide additional features and tools to assist with fraud detection, analytics, investigation, and response. Here are few additional benefits that Splunk can provide in the fight against fraud.
- Customized form-based dashboards with drilldowns to provide analysts easy access to data that is targeted to their investigative needs. This can allow lower tier analysts to perform searches over relevant data without learning the Splunk search language.
- Lookup tables and GUI-based editors to allow teams to easily manage and update their lookups that are used to enrich data or to maintain blacklists and whitelists.
- Summary dashboards to provide high-level overviews, trend analysis statistics and workflow based reports.
- Ease and flexibility in on boarding of a wide variety of data sources. Regardless of whether the data is structured (database tables) or unstructured proprietary data sources, Splunk can be configured to ingest the data.
- Correlation of otherwise disjoined data sources: Splunk provides the ability to join distinct data sources together to provide insight into sequence-based transactions.
- Flexibility to integrate and export data to other systems via scripting, alert actions, and dynamic forms or drilldowns.
- The ability to pull historical reports for compliance requirements and to assist in financial crime investigations.
What's Next?
Unquestionably, static based rules can be highly effective in detecting fraud and typically have a quick development cycle. However, as fraudsters continue to adapt and utilize new methods, it will become increasingly important to utilize machine learning and data science algorithms in the fight against fraud. Detecting anomalies and outliers through machine learning, utilizing adaptive thresholds, and other advanced techniques are clearly the next wave in fraud detection and prevention, and of course Splunk has an app for that! Checkout the Machine Learning Toolkit.
Conclusion
Function1 has had the opportunity to implement Splunk as a fraud detection and analytics solution with several customers in the financial industry. In each situation, they have found Splunk to be a highly effective and efficient tool in the fight against fraud. Our customers have consistently reported high values for Splunk prevented loses, particularly in the areas of fraudulent applications and member account takeovers.
As always, thanks for reading! Please reach out if you would like to discuss how we could help your organization use Splunk to meet its fraud detection needs.
- Log in to post comments